What is Artificial Intelligence:
Artificial intelligence (AI) is the intelligence displayed by machines, in contrast to the natural intelligence displayed by humans and animals, which includes knowledge and empathy. The difference between the previous paragraphs and the last paragraphs is usually indicated by the selected summary. 'Strong' AI is often referred to as AGI (Artificial General Intelligence) while attempts to mimic 'natural' intelligence are called ABI (Artificial Biological Intelligence). AI's leading manuals describe the field as a study of "intelligent agents": any device that recognizes its environment and takes steps that increase its chances of successfully achieving its goals. In general, the term "artificial intelligence" is often used to describe machines (or computers) that mimic the functions of "understanding" people who associate them with the human mind, such as "learning" and "problem solving"
What does artificial intelligence mean?
The power of a digital computer or a computer-controlled robot to perform tasks that are often associated with intelligent creatures. The term is often used in a project of developmental programs given to human psychological processes, such as the ability to think, find meaning, integrate, or learn from past experiences. Since the advent of the digital computer in the 1940s, it has been shown that computers can be programmed to perform more complex tasks - for example, to obtain proof of mathematical discourse or to play chess - with greater efficiency. However, without further advances in computer speed and memory capacity, there are currently no programs that can transform a person into a wide range of domains or tasks that require a lot of everyday knowledge. On the other hand, some programs have reached the level of professionalism of professionals and specialists in performing specific tasks, so that the ingenuity of this limited concept is available in a variety of applications such as medical diagnostics, computer search engines, and visual or handwriting.
Today, AI is generally thought to refer to “machines that respond to regeneration associated with traditional responses from humans, empowered by human thinking, judgment, and purpose.” According to researchers Shubhendu and Vijay, these software programs "make decisions that often require a [human] level of expertise" and help people anticipate problems or face problems as they arise. As John Allen and I pointed out in the April 2018 paper, such programs have three characteristics that form the essence of artificial intelligence: purpose, ingenuity, and adaptability.
In the rest of this paper, I discuss these qualities and why it is important to make sure that each agreement is in harmony with basic human standards. Each aspect of AI has the potential to move civilization forward in sustainable ways. But without adequate protection or the inclusion of behavioral considerations, AI utopia can quickly turn into dystopia.
Now, What is Intelligence?
All but the simplest human behavior is given to intelligence, and the most complex insect behavior is not considered to be intelligent. What is the difference? Consider the behavior of the evil digger, Sphex ichneumoneus. When the female wasp returns to its burrow with food, it first puts it on the threshold, checks its intruders, and then, when the shore is clear, puts its food inside. The true nature of the wasp's natural habits is revealed when food is removed a few inches from the entrance of its hive during the interior: when it appears, it will repeat the whole process several times when the food is removed. Spying - which is not obvious in the case of Sphex - should give it the power to adapt to new circumstances.
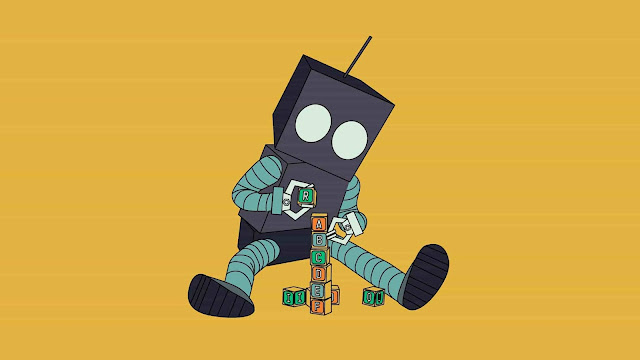
Psychologists often portray human wisdom in a single way and in the combination of many different skills. Research on AI focuses on the following areas of expertise: reading, consulting, problem-solving, recognition, and language use.
Artificial intelligence algorithms are designed to make decisions, usually using real-time data. It is not like artificial intelligence that can respond by machine or by predestination. Using sensors, digital data, or remote input, they gather information from a variety of sources, analyze information at once, and act on the information contained in that information. Therefore, they are designed for people on purpose and come to conclusions based on their quick analysis.
With significant improvements in storage systems, processing speed, and analytical techniques, these algorithms can become more complex in analysis and decision making. Financial algorithms can detect minute differences in stock ratings and perform market transactions that benefit from that information. The same principle applies to ecosystems that use sensors to determine whether a person is in a room and automatically adjust the temperature, cooling, and lighting based on that information. The aim is to save energy and use resources efficiently.
As long as these programs are in line with important human values, there is little risk of AI going to power or endangering people. Computers can be intentional while analyzing information in ways that add people or help them work at a higher level. However, if the software is poorly designed or based on incomplete or biased information, it could endanger humanity or replicate past injustices.
AI is usually done in conjunction with machine learning and data analysis, and emerging combinations enable powerful decision-making. Machine learning takes data and looks at the styles below. If it detects a specific problem, software developers can capture that information and use it to analyze the data to understand specific issues.
In consultation
Thinking of drawing indicators that are relevant to the situation. Indicators are classified as diminishing or diminishing. An earlier example is, “Fred has to be in a museum or a cafe. He is not in the restaurant; therefore it is in a museum, ”and lastly,“ Previous accidents of this kind were caused by metal failure; therefore this accident was caused by metal failure. ”The most striking difference between these types of assumptions is that in the case of a fact-finding fact the architectural truth confirms the veracity of the conclusion, and in the case of discovering the fundamental truth provides support for the conclusion without providing full proof. Deliberate consultation is common in science, where data is collected and developed models to try to predict and predict future performance — until negative data emerges forcing the model to be updated. Outline thinking is common in mathematics and logical reasoning, in which constructed extended structures of undeniable ideas from a small set of basic axioms and rules.
There has been a great deal of success in computer programming for imaginative drawing, especially diminished imaginary. Real thinking, however, involves more than just drawing directions; includes drawing that corresponds to the solution of a particular task or situation. This is one of the most difficult issues facing AI.
Adapting A.I.
The final quality that characterizes AI programs is the ability to learn and adapt as they combine information and make decisions. Active working intelligence should adjust as circumstances or circumstances change. This may include changes in financial circumstances, road conditions, environmental concerns, or war situations. AI must integrate these changes into its algorithms and make decisions on how to adapt to new opportunities.
One can picture these problems in a very significant way in the transportation environment. Private vehicles may use machine-to-machine communication to notify other vehicles on the road about impending traffic, potholes, road construction, or other roadblocks. Cars can take advantage of the experience of other cars on the road, without human involvement, and all of the combinations of their fast-track “experience” are completely transferred to other vehicles arranged in the same way. Their advanced algorithms, sensors, and cameras add information to current performance, and they use dashboards and visual mirrors to present real-time information so that human drivers can make sense of continuous traffic and vehicle conditions.
The same concept applies to AI designed for scheduling appointments. Some digital personal assistants can verify personal preferences and respond to email requests for personal appointments in a powerful way. Without human intervention, a digital assistant can make appointments, adjust schedules, and pass on your interests to other people. Creating flexible learning systems as they move has the potential to improve efficiency and effectiveness. These types of algorithms can handle complex tasks and make judgments that replicate or exceed what a person can do. But making sure they “learn” in fair and just ways is a very important thing for programmers.
Problem Solving
Problem-solving, especially in artificial intelligence, can be identified as a systematic search for possible types of actions to achieve the aforementioned goal or solution. Problem-solving strategies divide them into a special purpose and a common purpose. The special-purpose method is designed for a specific problem and usually uses certain features of the situation in which the problem is embedded. In contrast, the standard approach works for a variety of problems. One common objective method used in AI is to analyze methods — step-by-step, or incremental, to reduce the difference between the current situation and the ultimate goal. The program selects actions from a list of routes - in the event of a simple robot, this may include PICKUP, PUTDOWN, MOVE FORWARD, MOVE BACK, MOVE LEFT, and MOVE RIGHT - until the goal is reached.
Many different problems have been solved by artificial intelligence systems. Some examples find winning (or moving sequences) in a board game, establishing mathematical evidence, and deceiving “material things” in a computer-generated world.
Different Views
By observing nature it is scanned by various sensory organs, real or non-artificial, and the scene is divided into different objects in different spatial relationships. The analysis is complex in that the object may appear different depending on the angle at which it is viewed, the direction and power of lighting in the scene, and how the object contrasts with the surrounding field.
At present, the concept of artificial insemination is advanced enough to enable sensors to detect human sightings, autonomous vehicles to drive at a moderate speed on the open road, and traffic lights in buildings that collect empty cans of soda. One of the first programs to integrate comprehension and innovation was FREDDY, a stand-alone television-powered robot with an auxiliary hand, built at the University of Edinburgh, Scotland, during the 1966-73 era under Donald Michie. FREDDY was able to see a variety of objects and could be taught to combine simple art objects, such as a toy car, into a pile of random objects.
Language algorithms
A language is a system of symbols that has a description of a meeting. In this sense, language does not need to be limited to the spoken word only. Traffic signs, for example, form a minor language, it is a matter of convention that ⚠ means “danger ahead” in other countries. It varies in language that parts of the language have a meaning to the assembly, and the meaning of the language is very different from the so-called natural meaning, which is reflected in expressions such as "Those clouds mean rain" and "Decreased pressure means the valve is not working properly."
An important aspect of fully human languages - unlike bird calls and road signs - is their productivity. Productive language can form a variety of sentences.
It is easy to write computer programs that seem capable, in very restrictive situations, to respond fluently in human language to questions and statements. Although none of these programs fully understand the language, they can actually reach a point where their language command is inseparable from that of the average person. What, then, is involved in real understanding, if even a computer that uses language as a native speaker can be admitted to understanding it? There is no universally agreed-upon answer to this difficult question. In one sense, whether a person understands or not depends not only on a person's behavior but also on his or her history: to be understood, he or she must have learned the language and trained to take his or her place in the language of communication with other language users.
Global benefits of A.I.
There is no easy answer to that question, but programmers should incorporate key values into algorithms to ensure that they are relevant to people's concerns and to learn and adapt to methods that conform to social norms. This is why it is important to ensure that AI ethics are taken seriously and are embedded in community decisions. To maximize positive results, organizations should employ ethics and decision-makers in companies and software developers, have an AI code of conduct that sets out how various issues will be handled, set up an AI review board that regularly answers company ethics questions, and have audit trails for AI that demonstrates how various coding decisions are made, uses AI training programs for employees to apply ethical thinking in their daily work, and provides remedial measures where AI solutions harm or harm individuals or organizations.
With these types of security, communities will increase the likelihood that AI programs are deliberate, intelligent, and flexible while still in line with basic human values. That way, countries can move forward and reap the benefits of artificial intelligence and emerging technologies without sacrificing the essential qualities that define humanity.
Artificial Intelligence Objectives
AI research follows two different, competing methods, symbolic methods (or "top-down"), and a connection method (or "bottom-up"). The up-and-down approach seeks to replicate intelligence by analyzing independent understanding in the structure of the brain, by processing signals — where the label is symbolic. The downward path, on the other hand, involves creating neural networks of imitation by mimicking the structure of the brain — where the label is connected.
To illustrate the difference between these methods, consider the process of creating a system, which is equipped with a visual scanner, which recognizes letters. The down-to-top approach involves artificial network training to introduce characters to each other, slightly improving performance by "fixing" the network. (Adjustment corrects the response of various neural pathways and various motives.) In contrast, the downward approach involves writing a computer program that compares each character with geometric meanings. Simply put, neural functions are the basis of the upper extremity, and the figurative meanings are the basis of the upper extremity.
In The Fundamentals of Learning (1932), Edward Thorndike, a psychologist at Columbia University, New York City, first suggested that human learning contains a mysterious link between the nerves in the brain. In The Organization of Behavior (1949), Donald Hebb, a psychologist at McGill University, Montreal, Canada, suggested that learning involves mainly strengthening certain patterns of neural activity by increasing the chances of (weight) shooting neurons between parallel connections. The idea of a meaningful connection is described in the next section, Connectionism.
In 1957 two powerful advocates of symbolic AI - Allen Newell, a researcher at RAND Corporation, Santa Monica, California, and Herbert Simon, a psychologist and computer scientist at Carnegie Mellon University, Pittsburgh, Pennsylvania - summarized how to look up what they called a symbol of physical symptoms. This hypothesis states that the processing of signals is sufficient, essentially, to produce artificial intelligence in a digital computer and that, moreover, human intelligence is the result of the same kind of symbolic deception.
During the 1950s and 60s, the top and bottom methods were followed simultaneously, and both achieved remarkable results, if limited. During the 1970s, however, lower AI was ignored, and it was not until the 1980s that this trend came to the fore again. These days both methods are followed, and both are accepted as challenging. The metaphorical techniques work in simplified environments but are usually ruined when confronted with the real world; meanwhile, low-income researchers have failed to replicate very simple biological sensory systems. Caenorhabditis elegans, the most widely studied larvae, have about 300 nerves with their well-known means of communication. However, the link beauties have failed to mimic even this caterpillar. Clearly, neurons in connection education are far superior to the real thing.
History of A.I.
The first major work in the field of artificial intelligence was carried out in the mid-20th century by Alan Mathison Turing, a British logician. In 1935 Turing described a mysterious computer machine that contains unlimited memory and a scanner that goes back and forth with memory, sign by mark, reading its findings, and writing other symbols. Scanner actions are told by a command system that is stored in memory in the form of symbols. This is the concept of the stored Turing system, and its inclusion may be that the machine works, and modifies or upgrades its own system. Turing's pregnancy is now known simply as the Turing machine in general. All modern computers are actually Turing universal machines.
During World War II, Turing was a leading cryptanalyst leader in Government Code and Cypher School in Bletchley Park, Buckinghamshire, England. Turing was unable to turn to the project to build an electronic device that was kept inside the system until the end of hostilities in Europe in 1945. However, during the war, he gave serious thought to the issue of mechanical intelligence. One of Turing's colleagues in Bletchley Park, Donald Michie (founder of the Department of Intelligence and Understanding at the University of Edinburgh), later recalled that Turing often discussed how computers could learn information and solve new problems through guiding principles - a process now known as problem-solving.
Turing gave the first public talk (in London, 1947) to describe computer intelligence, saying, "What we need is a machine that can learn from experience," and that "the opportunity to let the machine change its commands gives way to this." In 1948 he introduced many central AI concepts in a report entitled “Intelligent Machinery.” However, Turing did not publish the paper, and many of his ideas were revived by others. For example, one of Turing's original ideas was to train a network of artificial neurons to perform specific tasks, a technique described in the Connectionism section.
A.I. At work
Small AI: Sometimes referred to as "Weak AI," this type of artificial intelligence works within a limited range and mimics human intelligence. Small AI tends to focus on doing one job very well and while these machines may seem smarter, they work under more challenges and limitations than human basic intelligence.
Artificial General Intelligence (AGI): AGI, sometimes referred to as "Powerful AI," is the kind of artificial intelligence we see in movies, such as robots from Westworld or Data from Star Trek: The Next Generation. The AGI is a generic machine that, much like a human being, can use that intelligence to solve any problem.
Machine and In-Depth Learning
Most Small AI is powered by advances in machine learning and in-depth learning. Understanding the differences between artificial intelligence, machine learning, and in-depth learning can be confusing. Venture capital Frank Chen offers a good idea of how to differentiate between them, note:
Simply put, machine learning consumes computer data and uses mathematical techniques to help it "learn" how to progress further in the workplace, without planning for that task, eliminating the need for millions of lines of written code. Machine learning consists of both supervised reading (using labeled data sets) and unchecked reading (using non-labeled data sets).
In-depth learning is a form of machine learning that uses inputs through the formation of neural-stimulated neural networks. Neural networks contain many hidden layers in which data is processed, allowing the machine to penetrate "deep" into its learning, enabling communication and instilling weight for excellent results.
“We must address, individually and collectively, moral and ethical issues raised by cutting-edge research in artificial intelligence and biotechnology, which will enable significant life extension, designer babies, and memory extraction.” —Gray Scott
The conclusion
In short, there have been some remarkable advances in recent years in the ability of AI programs to incorporate purpose, ingenuity, and adaptability into their algorithms. Instead of being a mechanic or specifying how machines work, AI software learns as it progresses and incorporates real-world knowledge into its decision-making, that's all. If you have any questions about our article please put it below, your response will be notified.
Also Read:
0 Comments
Thanks for your feedback.